Beyond Data Mesh and Data Fabric
Why Federated AI is the Pragmatic Future of Data Collaboration
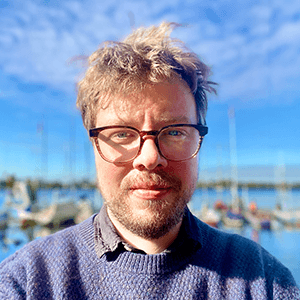

Listen to this article
Share
In recent years, Chief Data Officers (CDOs) and data leaders have faced a central challenge: how to get meaningful, analytics-ready data at scale without causing massive delays, operational friction, or compliance nightmares. Two architectural paradigms—data mesh and data fabric—have emerged as leading approaches to address these problems. Both aim to break down data silos, improve data accessibility, and drive faster insights. Yet, they come with their own complexities, especially when dealing with strict regulatory environments, global operations, and soaring costs of data harmonization.
Federated AI offers another way of working with distributed data that tackles a stubborn category of problems that data mesh or data fabric approaches may not fully resolve.
Exploring The Two Leading Architectures: Data Mesh and Data Fabric
Data mesh decentralizes data ownership. Instead of pushing data into a monolithic data lake or warehouse, each business domain (e.g., manufacturing, logistics, finance) creates and manages its own “data products.” This domain-oriented approach makes data more trustworthy and directly usable by the teams who understand it best, speeding innovation and reducing backlogs. It’s an excellent fit for CDOs who need to scale data initiatives and give domain teams autonomy. However, the mesh can’t solve every puzzle; it still relies on some level of data alignment and often runs into hurdles when data is sensitive, multi-regional, or bound by complex regulatory frameworks.
Data fabric, on the other hand, relies more on automation and metadata-driven integration. It provides a unified, virtualized view of all data sources—no matter where they’re stored—allowing analysts and data scientists to discover and query data seamlessly. For CDOs seeking quick wins and a stable, technology-driven foundation for analytics, a data fabric reduces the weariness of data integration and speeds up insights. However, when strict legal constraints prohibit even the virtual pooling or movement of certain sensitive datasets, a data fabric approach can still hit serious roadblocks.
The Hidden Gaps: Regulatory, Operational, and Cost Barriers That Slow Data Strategies
Despite their strengths, data mesh and data fabric can still leave data leaders struggling when:
Compliance issues block data movement: Regulatory frameworks like GDPR, HIPAA, export control, and dual-use restrictions, or country-specific laws like China’s Data Security Law (DSL), can forbid any form of centralizing or even virtually unifying data.
High costs of data harmonization: Even with mesh or fabric, it can take enormous time and money to “harmonize” data sets—agreeing on schemas, formats, links, and ingestion processes. These large-scale data projects consume months, if not years.
Data locked behind borders: For truly global insights, organizations need to combine knowledge from multiple regions. Cross-border data movement can be near-impossible due to conflicting legal standards, making global analytics and AI unreachable.
In such scenarios, adopting a data mesh or data fabric alone may help up to a point—but when you literally cannot move data, or the data integration costs become prohibitive, you need a more direct solution.
Federated AI Explained: Driving Insights at the Source, Without Moving Your Data
This is where Federated AI comes into play. Federated AI reverses the usual logic of data management and machine learning. Instead of bringing data to a model, it brings the models directly to the data sources—no matter how distributed or regulated they are.
Key Benefits of Federated AI: Speed, Savings, and Security Under Stricter Compliance
No Data Movement: Federated AI trains models locally at each data source and only shares model updates—no raw data moves. This preserves compliance with stringent regulations that prohibit cross-border data transfer or keep sensitive data locked in its original environment.
Cost Savings through Simplicity: By cutting out the complex, expensive data harmonization projects, you save significant resources. Training models directly at the source means you don’t need massive ETL pipelines or lengthy negotiation over schemas. The complexity and overhead of aligning multiple, often reluctant, data sources drops dramatically.
Speed of Impact: Instead of spending months or years trying to centralize or unify data, Federated AI allows you to start gleaning insights right away. It accelerates the path from data to actionable intelligence, unlocking value while abiding by every compliance and security requirement.
Built-in Security and Privacy: Because Federated AI never centralizes raw data, the risk profile changes. There’s no single “target” of sensitive information. Data remains secure in its original location, and only model parameters move, which are encrypted and governed by advanced security protocols.
Proven Success with Siemens: A Federated AI Case Study
Early adopters like Siemens have achieved outstanding results with Federated AI. In one leading plant, the first pass yield increased by 1.28%, while data transfer costs were slashed by 99.9% compared to centralized solutions. Model performance saw a remarkable boost, with the F1 score improving from 84% to 99.2% and recall rising from 77% to 95%. This significantly reduced the need for manual inspections and enhanced overall model accuracy. Additionally, Siemens achieved an 80% reduction in MLOps complexity compared to cloud-based solutions. For more details, explore our case study on revolutionizing PCB quality control.
Why You Should Consider Federated AI Now
Reduce Costs Dramatically: Stop funding massive data pipelines and harmonization projects. With Federated AI, you gain insights faster and cheaper.
Unlock Global Insights Under Strict Laws: Comply effortlessly with different jurisdictions. Whether it’s GDPR or complex export control laws, Federated AI lets you derive cross-border intelligence while keeping data in place.
Speed to Impact: Instead of spending your time building perfect pipelines, start iterating AI models right away. Your domain teams can innovate immediately, guided by the insights Federated AI delivers.
Partner with Katulu: Harness Federated AI to Accelerate Your Data Advantage
As a leader navigating the complexities of modern data landscapes, you want agility, compliance, and immediate ROI. Katulu’s Federated AI platform enables you to break free from the old assumption that all data must be unified or moved. Instead, deliver actionable AI insights at the source, reducing both cost and risk.
If you’re ready to overcome the limitations of data mesh and data fabric approaches in highly regulated, globally distributed environments, it’s time to consider Federated AI. With Katulu, you can innovate at the speed of insight—without ever compromising on privacy, security, or compliance.
Schedule a meeting with Katulu to learn more.
Drive Innovation with Confidence: Explore how our Federated AI Platform can help you solve your most pressing enterprise AI challenges.
Ready to go beyond barriers? Start with a free evaluation.
Start Building